AI AND MACHINE LEARNING IN DRUG DISCOVERY AND DEVELOPMENT
The pharmaceutical sector has witnessed a remarkable surge in data digitalisation in recent years, enabling ground-breaking advancements in drug discovering and development. However, this digital transformation introduces challenges in data acquisition, analysis, and application for solving complex clinical problems.
-1.png?width=1920&height=1080&name=ambridge%20(1)-1.png)
AI has emerged as a critical enabler, offering the capability to process vast datasets efficiently and provide actionable insights, significantly advancing the pharmaceutical landscape.
THE NEW ROLE OF AI IN THE PHARMACEUTICAL INDUSTRY
AI leverages advanced systems and networks that mimic human intelligence to interpret and learn from data, enabling independent decision-making for specific objectives. So the BIG question, will AI take over jobs? Well while AI enhances efficiency, it complements rather than replacing human expertise completely. It’s applications in pharmaceuticals range from drug design and personalised medicine to clinical data management and strategic decision-making. For example, E-VAI, an AI platform by Eularis, uses machine learning (ML) to generate analytical roadmaps, aiding pharmaceutical marketing by predicting sales drivers and optimising resource allocation.
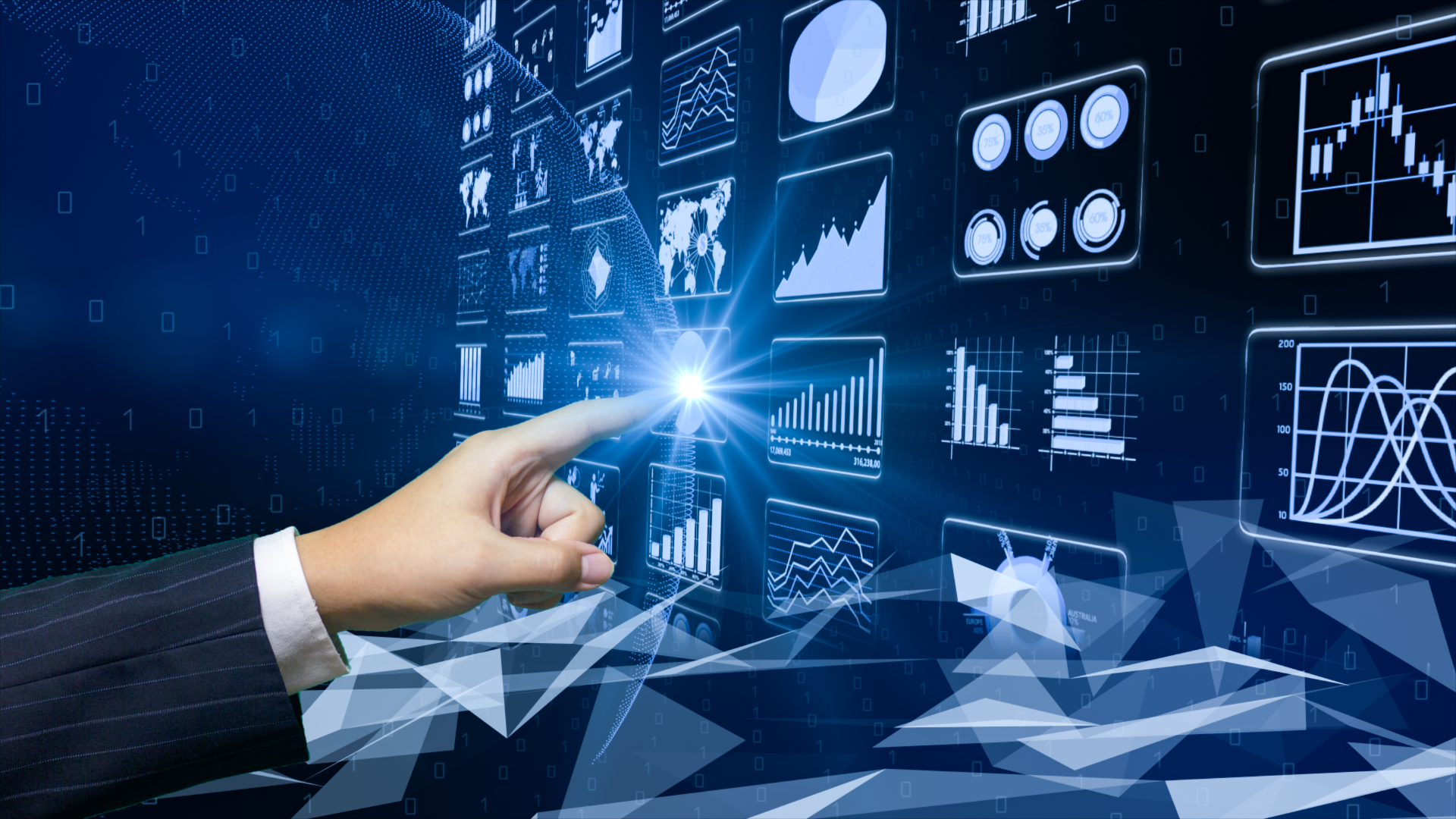
the common challenges in ai implementation
So despite its transformative potential, AI still faces significant challenges in the pharmaceutical domain, including:
- Data Complexity: Drug development involves massive, diverse, and uncertain datasets, such as millions of compounds, which traditional ML tools struggle to process.
- QSAR Model Limitations: Quantitative structure-activity relationship (QSAR) – based models are limited in predicting complex biological properties like efficacy and adverse effects. They are hindered by small training sets, experimental errors, and lack of validation.
However recent advancements in AI, including deep learning (DL) and big data analytics, are addressing these challenges. For instance, a 2012 Merck-sponsored QSAR ML challenge highlighted DL’s superior predictivity for 15 ADMET datasets, a critical aspect of drug candidate evaluation. The events of this experiment are laid in more detail in this report, on page 5. IJCRT24A4690.pdf
IMPACT ON THE LAB DESIGN AND BUILD INDUSTRY
The integration of AI and machine learning in drug discovery is reshaping the requirements and priorities for lab design and construction. Modern laboratories must now accommodate advanced AI-driven workflows, including high-throughput data analysis, automated robotics, and integrated multiomic sequencing technologies. This demands infrastructure tailored to support cutting-edge computational tools, flexible layouts for evolving research needs, and robust data management systems.
Additionally, labs must prioritise collaboration spaces that promote interdisciplinary work between data scientists, biologists, and chemists, reflecting the convergence of traditional wet-lab activities with AI-enhanced analytics. By aligning lab designs with these emerging needs, the industry enables researchers to harness AI’s full potential, accelerating innovation and enhancing productivity.
-1.png?width=1920&height=1080&name=ambridge%20(4)-1.png)
AI Applications in drug discovery
AI significantly enhances several key aspects of drug discovery:
- Physicochemical Property Prediction:
- Tools powered by ML predict properties like solubility, ionisation, and permeability, which influence pharmacokinetics.
- Algorithms analyse molecular descriptors, such as SMILES strings and 3D atom coordinates, to generate feasible molecules.
- Drug-Target Binding Affinity (DTBA):
- Accurate prediction of DTBA is vital for assessing drug efficacy and toxicity.
- Feature-based and similarity-based AI methods predict DTBA by analysing drug and target chemical moieties or similarities.
- Web applications like ChemMapper and SEA utilize these approaches, complemented by ML strategies like KronRLS and SimBoost.
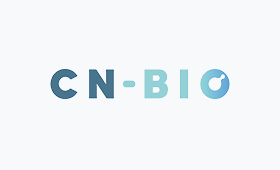
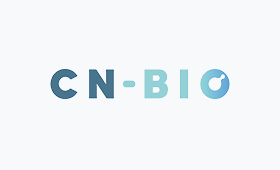
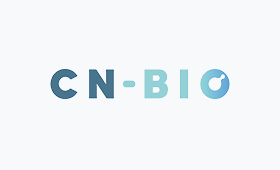
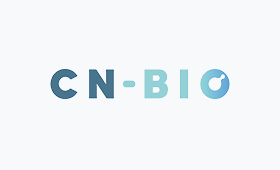
TARGET IDENTIFICATION AND VALIDATION
AI facilitates the identification of promising drug targets by analysing genomics, proteomics, and clinical data.
Approaches include:
- Statistical Analysis: Employing genome-wide association studies (GWAS) to uncover candidate genes.
- Network-Based Methods: Exploring gene co-expression and disease networks.
- ML Techniques: Predicting drug targets using classifiers and regression models.
Companies like Evotec and Exscientia exemplify AI’s potential, with Exscientia’s “Centaur Chemist” platform identifying an anti-cancer molecule in just eight months.
-1.png)
advancements in sequencing technologies
Nanopore sequencing represents a transformative technology in drug discovery, addressing limitations of legacy short-read sequencing methods. Its features include:
Ultra-rich multiomic data: Providing comprehensive insights into health, disease mechanisms, and drug action.
Versatility: Capable of sequencing DNA/RNA fragments of any length without amplification bias.
Real-time analysis: Delivering actionable results promptly.
Oxford Nanopore’s platform exemplifies these advantages, enabling novel target identification, biomarker discovery, and quality control during therapeutic manufacturing.
-1.png?width=1920&height=1080&name=ambridge%20(2)-1.png)
AI and machine learning are revolutionizing drug discovery and development, offering unprecedented capabilities in data analysis, drug design, and clinical decision-making. While challenges persist, innovations in deep learning, target validation, and sequencing technologies promise to overcome these barriers, expediting the journey from bench to bedside.
As AI continues to evolve, its integration into pharmaceutical processes will redefine the boundaries of possibility, improving patient outcomes and reshaping the future of medicine.
READ OUR WORKPLACE GUIDE
HOW MUCH DOES A COMMERCIAL FIT-OUT COST IN 2024?
In 2024, the overall cost of an office fit out will vary depending on a variety of factors including size and scope, materials used, labour costs and finishes. Companies may also encounter unexpected costs related to site access and regulatory requirements. Planning ahead can help manage the costs of a commercial fit out as this will allow for research into suitable materials and suppliers, meaning it is important to do detailed research before making any decisions.
.png)
LET'S TALK ABOUT YOUR NEXT REQUIREMENT